- Flipped.ai Newsletter
- Posts
- AI learns table tennis from DeepMind
AI learns table tennis from DeepMind

Transform your hiring with Flipped.ai – the hiring Co-Pilot that's 100X faster. Automate hiring, from job posts to candidate matches, using our Generative AI platform. Get your free Hiring Co-Pilot.
Dear Reader,
Flipped.ai’s weekly newsletter read by more than 75,000 professionals, entrepreneurs, decision makers and investors around the world.
In this newsletter, we highlight a groundbreaking achievement by Google DeepMind, which has successfully trained a robot to play table tennis at an amateur human level. This marks the first time a robot has competed in a sport with such proficiency. We'll explore the challenges, innovative methods, and potential future implications of this technological milestone.
Before, we dive into our newsletter, checkout our sponsor for this newsletter.

A Gold IRA can diversify your portfolio and safeguard your retirement
Safeguarding your retirement with a Gold IRA can help you shield your wealth from market shifts, economic uncertainty, and inflation. And with gold’s value projected to increase in 2024, now is a good time to invest. Planning for retirement involves more than saving, so fortify your portfolio with gold today and plan for a better tomorrow.
See our list of Best Gold IRA Companies to start planning a better retirement.
Google DeepMind's Table Tennis Robot: A leap towards human-level robotic performance

Source: Google DeepMind
In a remarkable display of technological prowess, Google DeepMind has achieved a significant milestone in the field of robotics and artificial intelligence (AI). The company successfully trained a robot to play table tennis at an amateur human level, marking the first time a robot has been taught to compete in a sport with humans at such a level of proficiency. This article delves into the details of this groundbreaking achievement, exploring the challenges, methodologies, implications, and future potential of this technological innovation.
The intersection of robotics and sports
Robotics has made significant strides in recent years, with robots taking on increasingly complex tasks, from industrial automation to sophisticated surgical procedures. However, the ability to perform in dynamic, unpredictable environments has remained a significant challenge. Sports, with their need for real-time decision-making, adaptability, and physical dexterity, present a particularly demanding arena for robotic performance.
Google DeepMind, renowned for its work in AI and machine learning, has taken on this challenge by developing a robot capable of playing table tennis at a competitive level. This achievement is not just a technological novelty; it represents a crucial step towards creating robots that can perform useful tasks skillfully and safely in real-world environments, such as homes, workplaces, and beyond.
The genesis of the Table Tennis Robot
Background and motivation
The inspiration for this project stemmed from the desire to push the boundaries of what AI and robotics could achieve in dynamic, real-world scenarios. While AI has mastered strategic games like chess and Go, which rely heavily on decision-making and strategy, physical sports introduce additional layers of complexity. Table tennis, in particular, was chosen because it requires not only strategic thinking but also speed, precision, and the ability to adapt quickly to an opponent's actions.
Google DeepMind's researchers were motivated by the challenge of creating a robot that could compete with humans in a physical sport, something that had never been done at this level before. The project aimed to test the limits of AI in dynamic environments and explore the potential applications of these advancements in various industries.
Initial challenges
Training a robot to play table tennis posed several significant challenges. Unlike strategic games that can be simulated in a controlled environment, table tennis requires real-time interactions with a rapidly changing environment. The robot needed to be able to track the ball's movement, anticipate its trajectory, and respond quickly and accurately—all while adapting to the playing style of its human opponent.
Moreover, the physical constraints of the robot, such as the speed and precision of its movements, added another layer of difficulty. The researchers had to ensure that the robot could move its paddle quickly enough to return fast serves, position itself correctly to handle high or low balls, and avoid collisions that could damage the equipment.
The technology behind the robot
AI and Machine Learning Techniques
The core of the table tennis robot's capabilities lies in its advanced AI and machine learning algorithms. The robot was trained using a combination of reinforcement learning and neural networks, two powerful techniques that have been instrumental in many of DeepMind's past successes.
Reinforcement learning: This technique allows the robot to learn from its interactions with the environment by receiving feedback in the form of rewards or penalties. For instance, successfully returning a ball or winning a point would result in a positive reward, while missing a shot or losing a point would result in a penalty. Over time, the robot learns to maximize its rewards by improving its performance.
Neural networks: The robot's decision-making processes are powered by neural networks, which mimic the way the human brain processes information. These networks enable the robot to analyze complex data, such as the speed, spin, and trajectory of the ball, and make real-time decisions about how to respond.
Simulation-based training
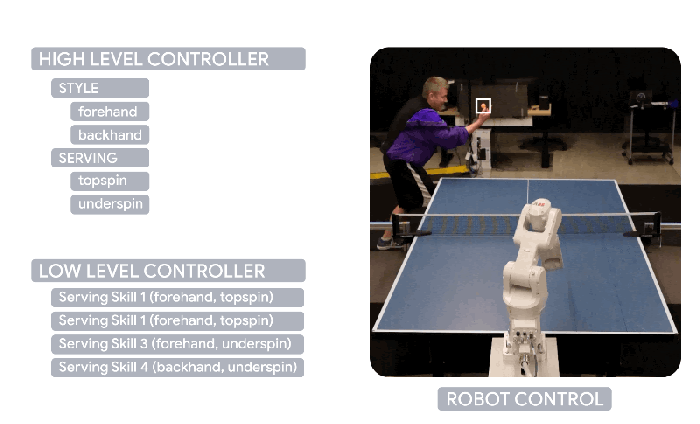
Source: Google DeepMind
Before being deployed in real-world matches, the robot was trained extensively in a simulated environment. This simulation was designed to accurately reflect the physics of table tennis, allowing the robot to practice its skills in a controlled setting.
The researchers compiled a comprehensive dataset of table tennis ball states, including information on the ball's position, speed, and spin. This data was used to create a virtual environment where the robot could practice returning serves, hitting forehand topspin shots, and executing backhand returns.
By training in this simulated environment, the robot was able to master the fundamental skills required for table tennis without the limitations of real-world constraints, such as equipment wear and tear or the need for human opponents. This approach also allowed the researchers to rapidly iterate on the robot's training, testing different strategies and refining the AI algorithms.
Real-world deployment and fine-tuning
Once the robot had demonstrated proficiency in the simulated environment, it was deployed in real-world matches against human opponents. During these matches, the robot collected data on its performance, including how it responded to different types of shots and how well it adapted to the playing styles of its opponents.
This real-world data was then fed back into the simulation, allowing the robot to refine its skills further. This continuous feedback loop—where the robot's performance in real matches informs its training in simulation—was critical to the robot's success. It enabled the AI to adapt to new challenges and improve its performance over time.
The robot’s performance: Matches against human players
Results and achievements
The table tennis robot's performance in real-world matches was impressive, particularly given the complexity of the task. The robot played 29 full games against human opponents of varying skill levels, winning 13 of them. This included victories against all beginner-level players and 55% of the matches against intermediate-level opponents.
These results demonstrate that the robot has achieved a solid amateur-level performance, a significant accomplishment given the challenges involved. While the robot struggled against advanced players—losing all of its matches against them—it still managed to hold its own in many games, showcasing its ability to adapt and compete in a dynamic environment.
Strengths and limitations
The robot's strengths were evident in its ability to execute basic table tennis skills, such as returning serves and adapting its playstyle based on its opponent's actions. These are critical skills for success in table tennis, and the robot's mastery of them is a testament to the effectiveness of its training process.
However, the robot also faced several limitations. It struggled with high balls, fast serves, and shots with heavy spin—challenges that are likely due to the physical constraints of the robotic arm and the complexity of processing real-time data in such a fast-paced environment. The robot's decision-making algorithms also have room for improvement, particularly in anticipating and countering more sophisticated strategies from human opponents.
Challenges and technical hurdles
Difficulties with certain shots
One of the key challenges faced by the robot was its difficulty in handling specific types of shots, particularly high balls and fast serves. These shots require precise timing and positioning, and the robot's current hardware and software systems are not yet fully optimized to handle them.
For instance, high balls posed a challenge because they required the robot to adjust its positioning and angle its paddle correctly to return the shot. If the ball was too high, the robot often struggled to reach it in time, resulting in a missed shot. Similarly, fast serves required the robot to react quickly and accurately, which was difficult given the limited processing time available.
These challenges highlight the limitations of the robot's current capabilities and underscore the need for further research and development to improve its performance in these areas.
Hardware limitations and data constraints
In addition to the challenges posed by specific types of shots, the robot also faced limitations related to its hardware and the availability of data. The robot's physical components, including its arm and paddle, are subject to mechanical constraints that can affect its performance. For example, the robot's arm may not be able to move as quickly or accurately as a human arm, limiting its ability to execute certain shots.
Furthermore, the amount of data available for training the AI is also a limiting factor. While the robot has access to a large amount of data from its simulated training, the real-world data is more limited. This can affect the AI's ability to learn and adapt to new situations, as it may not have encountered certain scenarios during its training.
Ethical considerations and human-robot interaction
Ethical implications of AI in sports
As AI continues to advance, it is essential to consider the ethical implications of deploying robots in human environments. While the table tennis robot is primarily a research project, its success raises important questions about the future of AI and robotics in society.
One key ethical consideration is ensuring that robots are designed and programmed to operate safely and responsibly. This includes not only ensuring that robots do not pose a physical threat to humans but also addressing concerns related to privacy, data security, and the potential for AI to be used in ways that could harm individuals or communities.
For example, as robots become more capable of interacting with humans, there is a risk that they could be used to monitor or control individuals in ways that infringe on their privacy or autonomy. Ensuring that AI is used in ways that respect human rights and promote the common good is a critical challenge for researchers and policymakers.
The future of human-robot interaction
The table tennis robot's ability to engage with humans in a social and recreational context also raises interesting questions about the future of human-robot interaction. As robots become more capable of participating in activities traditionally reserved for humans, there is potential for them to play a role in social and recreational settings.
Hire top-tier Indian tech talent affordably

Powered by Flipped.ai
Are you looking to build a high-caliber tech team in India without breaking the bank? Look no further than SourceTalent.ai, powered by Flipped.ai, your smartest candidate sourcing platform!
SourceTalent.ai offers a revolutionary approach to hiring, leveraging AI-powered technology to connect you with the best tech talent in India, all at competitive rates.
Key features to enhance your hiring process
Instant recommendations: Access a database of over 24 million Indian candidates and receive instant recommendations tailored to your hiring needs.
AI-powered candidate matching: Our advanced AI algorithms match and rank candidates based on your job requirements, saving you time and effort in the screening process.

Automated hiring process: Utilize our suite of AI-powered tools to automate job description generation, screening questions, candidate recommendations, and more, streamlining your entire hiring workflow.
Comprehensive candidate assessment: Conduct video-based interviews with unlimited candidates using our innovative video interview platform. Generate interview questions, send invites seamlessly, and gain deeper insights into your candidates' abilities.

Why SourceTalent?
Affordable excellence: Benefit from competitive pricing starting at Rs400 / $5 per job posting.
Quality candidates: Access a diverse pool of talented tech professionals in India.
Streamlined recruitment: Conduct efficient and insightful video assessments at a minimal cost.
Flexible solutions: Ideal for startups and multinational corporations alike.
Indian talent available globally
US companies can also benefit from our platform to access India's exceptional tech talent pool at competitive rates!
Sign up with SourceTalent.ai today and check out our special launch offer! Hire top-tier Indian tech talent faster, smarter, and more affordably than ever before.
Get started today

Visit SourceTalent.ai to explore how we can transform your hiring process. Unlock the power of AI-driven recruitment with SourceTalent.ai!
Click here to access SourceTalent: [Link]
Reach out to our team at [email protected] to explore how SourceTalent can transform your hiring process. Join us in embracing innovation and cost-effective recruitment solutions!
Stay updated with the latest AI insights—subscribe to this newsletter today!
Want to get your product in front of 75,000+ professionals, entrepreneurs decision makers and investors around the world ? 🚀
If you are interesting in sponsoring, contact us on [email protected].
Thank you for being part of our community, and we look forward to continuing this journey of growth and innovation together!
Best regards,
Flipped.ai Editorial Team